ITC Mobile Conference Guide
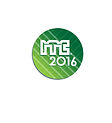
Wednesday Keynote Addresss 4:30
Special Keynote in Honor of Professor Edward J. McCluskey -
Special tributes to Professor McCluskey will be presented from 5:30 p.m. to 5:45 p.m.
Hardware Inference Accelerators for Machine Learning
Room Ballroom AB
Rob A. Rutenbar, Professor, University of Illinois at Urbana-Champaign
Machine learning (ML) technologies have revolutionized the ways in which we interact with large-scale, imperfect, real-world data. As a result, there is rising interest in opportunities to implement ML efficiently in custom hardware. We have designed hardware for one broad class of ML techniques: Inference on Probabilistic Graphical Models (PGMs). In these graphs, labels on nodes encode what we know and “how much” we believe it; edges encode belief relationships among labels; statistical inference answers questions such as “if we observe some of the labels in the graph, what are most likely labels on the remainder?” These problems are interesting because they can be very large (e.g., every pixel in an image is one graph node) and because we need answers very fast (e.g., at video frame rates). Inference done as iterative Belief Propagation (BP) can be efficiently implemented in hardware, and we demonstrate several examples from current FPGA prototypes. We have the first configurable, scalable parallel architecture capable of running a range of standard vision benchmarks, with speedups up to 40X over conventional software. We also show that BP hardware can be made remarkably tolerant to the low-level statistical upsets expected in end-of-Moore’s-Law nanoscale silicon and post-silicon circuit fabrics, and summarize some effective resilience mechanisms in our prototypes.
About the speaker: Rob A. Rutenbar received the Ph.D. degree from the University of Michigan, Ann Arbor, in 1984. From 1984 to 2009, he was faculty at Carnegie Mellon, where he held the Stephen J. Jatras (E’47) Chair in Electrical and Computer Engineering. In 2010 he joined the University of Illinois at Urbana-Champaign, where he is currently the Abel Bliss Professor and Head of Computer Science. His research has focused in three broad areas: tools for a variety of IC design problems; methods to manage the messy statistics of nanoscale chip designs; and custom silicon architectures for challenging tasks such as speech recognition and machine learning. His work has been featured in venues ranging from EETimes to the Economist magazine. He is a Fellow of the ACM and IEEE.